Is Noise the Key to Artificial General Intelligence?
Is Noise the Key to Artificial General Intelligence?
Converging evidence indicates noise plays a fundamental role in the brain.
Posted Jun 09, 2016
Let's take hearing as an example. We each have an auditory threshold, and if a sound is below this threshold we cannot hear it. However, if we add the right amount of noise to a weak sound it can often be detected by our brains. This is because our hearing threshold is a nonlinear detection device. This means that the output of the auditory neurons does not vary in a straightforward way with the input. The figure below illustrates the concept of stochastic resonance.
Left: a weak sine wave below the detection
threshold. Right: noise added to the wave boosts information about the
wave above the threshold.
Source: By Matt Hall - Own work
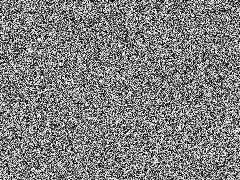
Source: By Jorge Stolfi - Own work, CC BY-SA 3.0
A fundamental conundrum that stochastic resonance addresses is the brute fact that noise is ubiquitous in the environment, biological systems and the brain. Given this fact, it would indeed fly in the face of what we know from evolution that biological systems would not have evolved adaptations to noise. A more efficient approach to handling noise in biological systems is perhaps exploiting it, as the theory of stochastic resonance predicts, rather than fighting it. Because of the robustness of stochastic resonance phenomenon in a wide variety of domains a key debate in neuroscience is whether the brain itself exploits internal neural noise via stochastic resonance. Because noise and signal are externally added in experimental settings, noise benefits in these studies are only direct evidence that the systems under investigation are nonlinear. A definitive demonstration of neural stochastic resonance in vivo remains elusive.
Advertisement
The fields of artificial intelligence and machine learning have in
recent years shifted focus from solving modality specific learning,
perceptual and motor problems, to working on general learning algorithms
that can handle data from any modality.
The goal of artificial general intelligence is to create an autonomous
agent that can actively interact with its environment, reason about its
own program code, and make improvements without the assistance of human
programmers. This type of system would require embodiment along with
perceptual sensors that mimic the behavior and information generation
capabilities of biological sensors. Stochastic resonance likely plays a
central role in bio-sensing as natural creatures must navigate in noisy
and uncertain environments. It is also possible that stochastic
resonance is exploited during cross-modality information fusion at the
level of conscious awareness. Artificial intelligence has returned to
its original ambitions of creating an artificial mind with general
adaptive intelligence.Even though the concepts of artificial neural networks are decades old, the next generation of neural-inspired approaches like reinforcement learning, deep learning and unsupervised feature learning have advanced computer vision, natural language processing, game playing and speech recognition. Reinforcement learning is a well-established theory of hippocampal memory formation and dopaminergic reward-based learning. DeepMind recently used a general deep learning and reinforcement learning architecture to develop a system that learned how to exceed human performance in video games with no training.
The recent advances in learning algorithms through neuroscientific insight have also returned the study of the brain to a prominent position in artificial intelligence. There is renewed optimism in machine learning and artificial intelligence that understanding how the brain solves intelligence will lead to rapid advances in machine intelligence (see commentary by Hassabis). Indeed the fields of artificial intelligence and neuroscience are developing a symbiotic relationship in which theoretical and empirical breakthroughs in one field can lead directly to breakthroughs in the other. Could the next breakthrough in either field come from stochastic resonance?
Advertisement
In parallel but largely independently of mainstream machine learning,
over the last twenty years within physics and related fields neural
network models have been developed that theoretically demonstrate the
plausibility of stochastic resonance as a mechanism by which nonlinear
threshold devices like neurons detect weak signals.
Furthermore, in model networks of coupled neuronal oscillators, noise
has been found to aid in the onset of network synchronization – a
phenomenon called coherence resonance.
This work is primarily carried out by physicists and electrical
engineers interested in studying how stochastic resonance and coherence
resonance affect synchronization phenomena in networks of nonlinear
dynamical systems.Interestingly, the beneficial role of noise has also long been recognized in machine learning, for example adding noise to synaptic weights enhances fault tolerance, generalization and the learning trajectory in multilayer perceptron learning. It is widely appreciated that additive noise improves learning and generalization performance in learning algorithms. This work has likely been inadvertently discovering evidence of stochastic resonance.
In biology, psychology and neuroscience evidence for stochastic resonance in human and animal central nervous systems is widespread. Background auditory noise improves cognitive performance in children with attention-deficit-hyperactivity disorder, possibly by modulating the gain function of dopamine neurons through stochastic resonance. The optimal auditory noise level induces synchronization within and between cortical sources of EEG activity when perceiving subthreshold tones. Ubiquitous cross-modal stochastic resonance effects have been observed in humans: auditory noise facilitates tactile, visual and proprioceptive sensations. Stochastic resonance has been demonstrated in binocular rivalry. Electrical noise improves the ability of the paddlefish in detecting plankton swarms. Kellogg and Tay showed that extrinsic noise allows populations of cells to entrain robustly under a wider range of inputs thus facilitating transcription control for gene expression. Noise may play a central role in consciousness and decision making.
In biomedical engineering, stochastic resonance has been found to reduce gait variability in the elderly and to help prevent falls. Additive noise enhances the performance of EEG-based augmented cognition systems. Stochastic whole body vibrations reduce symptoms of Parkinson’s disease.
The term "stochastic resonance" was first introduced in 1981 by Benzi as a mechanism by which random perturbations in Earth’s climate together with the eccentricity of its orbit cause the climate to switch from warm to cool phases in a 100,000 year cycle. While this explanation for the oscillations between ice ages and warm periods is still unproven, stochastic resonance has since been well-established in a huge variety of natural phenomena and nonlinear systems.
Learning algorithms based on reinforcement learning try to optimize reward. There exists a large literature modelling both dopaminergic reward-based learning and hippocampal memory formation using variants of reinforcement learning. Neurophysiologically inspired robotic cognitive control using reinforcement learning has been used to successfully handle environmental uncertainty. The DeepMind algorithm is directly inspired by hippocampal memory replay, which is thought to involve the sequential reactivation of hippocampal place cells that represent previously experienced behavioral trajectories. Interestingly, there are several models of dopamine function and hippocampal CA1 neurons that find evidence of stochastic resonance improving signal detection. Computational modelling has shown that dopaminergic neurons and of hippocampal neurons likely benefit from the presence of noise, either endogenously or from external sources.
The brain is still far better at many learning tasks compared to computers. If we can understand how the brain exploits stochastic resonance we may be able to improve machine learning. And conversely if we can use stochastic resonance to improve artificial learning algorithms we will be in a better theoretical position to solve how the brain uses noise. A convergence in these two lines of research, noise benefits and deep or reinforcement learning, could yield advances in both artificial intelligence and neuroscience. In fact, the DeepMind reinforcement learning algorithm introduces a novel feature that randomizes over the data, thereby removing correlations in the observation sequence and smoothing over changes in the data distribution. This kind of additive noise benefit might be further exploited by explicitly using the well-established mathematical concepts of stochastic resonance together with reinforcement learning. Future research should focus on synthesizing these lines of research to develop novel algorithms that are robust to uncertainty and take advantage of nature’s most plentiful untapped resource: noise.
References
Benzi R, Sutera A, Vulpiani A. (1981). The mechanism of stochastic resonance. J. Phys. A: Math. Gen. 14 L453 doi:10.1088/0305-4470/14/11/006 .
Brooks R, Hassabis D, Bray D, Shashua A. (2012). Turing centenary: Is the brain a good model for machine intelligence? Nature. 2012 Feb 22;482(7386):462-3. doi: 10.1038/482462a.
Carr M, Jadhav S, & Frank L. (2011). Hippocampal replay in the awake state: a potential physiological substrate of memory consolidation and retrieval. Nature Neuroscience, 14(2), 147–153. doi:10.1038/nn.2732.
Casson A. (2013). Towards Noise-Enhanced Augmented Cognition. Foundations of Augmented Cognition Lecture Notes in Computer Science 8027. 259-268 doi:10.1007/978-3-642-39454-6_27.
Du K, Swamy M. (2014). Neural Networks and Statistical Learning. Springer-Verlag London. doi: 10.1007/978-1-4471-5571-3.
Galica A, Kang H, Priplata A, D’Andrea S, Starobinets O, Sorond F, … Lipsitz L. (2009). Subsensory Vibrations to the Feet Reduce Gait Variability in Elderly Fallers. Gait & Posture. 30(3). 383–387. doi:10.1016/j.gaitpost.2009.07.005
Gong P, Xu J. (2001). Global dynamics and stochastic resonance of the forced FitzHugh-Nagumo neuron model. Phys Rev E Stat Nonlin Soft Matter Phys. 63(3 Pt 1):031906.
Kellogg R, Tay S. (2015). Noise Facilitates Transcriptional Control under Dynamic Inputs. Cell. 160, 381-392, doi:10.1016/j.cell.2015.01.013.
Khamassi M, Lallée S, Enel P, Procyk E, & Dominey P. (2011). Robot Cognitive Control with a Neurophysiologically Inspired Reinforcement Learning Model. Frontiers in Neurorobotics. 5, 1. doi:10.3389/fnbot.2011.00001
Kim Y, Grabowecky M, Suzuki S. (2006) Stochastic resonance in binocular rivalry. Vision Res. 46(3):392-406.
Kosko, B. (2006). Noise, Viking.
Li S, von Oertzen T, Lindenberger U. (2006). A neurocomputational model of stochastic resonance and aging. Neurocomputing. 69, Issues 13–15, 1553-1560, ISSN 0925-2312, doi:org/10.1016/j.neucom.2005.06.015.
Lindner B, Garcı́a-Ojalvo J, Neiman A, Schimansky-Geier L. (2004). Effects of noise in excitable systems. Physics Reports. 392. 6. 321-424, ISSN 0370-1573, doi:10.1016/j.physrep.2003.10.015.
Lugo E, Doti R, Faubert J. (2008). Ubiquitous Crossmodal Stochastic Resonance in Humans: Auditory Noise Facilitates Tactile, Visual and Proprioceptive Sensations. PLoS ONE 3(8): e2860. doi: 10.1371/journal.pone.0002860
McDonnell MD, Abbott D. (2009). What Is Stochastic Resonance? Definitions, Misconceptions, Debates, and Its Relevance to Biology. PLoS Comput. Biol. 5(5): e1000348. doi: 10.1371/journal.pcbi.1000348
McDonnell MD, Ward L. (2011). The benefits of noise in neural systems: bridging theory and experiment. Nature Reviews Neuroscience 12, 415-426. doi:10.1038/nrn3061
Mnih V, Kavukcuoglu K, Silver D, Rusu, Veness J, Bellemare M. G, Graves A, Riedmiller M, Fidjeland A. K, Ostrovski G, Petersen S, Beattie C, Sadik A, Antonoglou I, King H, Kumaran D,
Wierstra D, Legg S, Hassabis D. (2015). Human-level control through deep reinforcement learning.
Nature, vol. 518, p 1529, 26. doi:10.1038/nature14236
Moss F, Ward M, Sannita W. (2004). Stochastic resonance and sensory information processing: a tutorial and review of application. Clin. Neurophysiol. 115, 267-281. doi:10.1016/j.clinph.2003.09.014.
Murray A, Edwards P. (1994). Synaptic Weight Noise During MLP Learning Enhances Fault-
Tolerance, Generalization and Learning Trajectory. IEEE Transactions on Neural Networks. 5. 792-802.
Ngiam J, Khosla A, Kim M, Nam J, Lee H, Ng A. (2011). Multimodal Deep Learning. Proceedings of the Twenty-Eighth International Conference on Machine Learning
Nozaki D, Mar D, Grigg P, Collins, J. (1999). Effects of Colored Noise on Stochastic Resonance in Sensory Neurons. Phys. Rev. Lett. 82, 2402.
Perlovsky L. (2013). Learning in brain and machine—complexity, Gödel, Aristotle. Frontiers in Neurorobotics, 7, 23. doi:10.3389/fnbot.2013.00023.
Russell D, Wilkens L, Moss F. (1999). Use of behavioural stochastic resonance by paddle fish for feeding. Nature. 402. doi:10.1038/46279
Schmidhuber J. (2015). Deep Learning in Neural Networks: An Overview. Neural Networks, 61, 85-117 doi: 10.1016/j.neunet.2014.09.003.
Sikström S, Söderlund G. (2007). Stimulus-dependent dopamine release in attention-deficit/hyperactivity disorder. Psychol Rev. 114(4):1047-75.
Söderlund G, Sikström S, Smart A. (2007). Listen to the noise: noise is beneficial for cognitive performance in ADHD. J Child Psychol Psychiatry. 48(8):840-7.
Stacey W, Durand D. (2000). Stochastic resonance improves signal detection in hippocampal CA1 neurons. J Neurophysiol. 83(3):1394-402.
Stocks N. (2001). Suprathreshold stochastic resonance: an exact result for uniformly distributed signal and noise. Phys. Lett. A. 279, 308-312.
Ward L, Doesburg S, Kitajo K, MacLean S, Roggeveen A. (2006). Neural synchrony in stochastic resonance, attention, and consciousness. Can J Exp Psychol. 60(4):319-26.
Ward L, MacLean, & Kirschner A. (2010). Stochastic Resonance Modulates Neural Synchronization within and between Cortical Sources. PLoS ONE, 5(12), e14371. doi:10.1371/journal.pone.0014371
Wellens T, Shatokhin V, Buchleitner, A. (2004). Stochastic Resonance. Rep. Prog. Phys. 67 45 doi:10.1088/0034-4885/67/1/R02.
Wimmer G, Daw N, Shohamy D. (2012). Generalization of value in reinforcement learning by humans. European Journal of Neuroscience. 35. 1092–1104, doi:10.1111/j.1460-9568.2012.08017.x
Wise R. (2004). Dopamine, Learning and Motivation. Nat. Rev. Neuro. 5. 483-494. doi:10.1038/nrn1406.
Xu X, Zuo L, Huang Z. (2014). Reinforcement learning algorithms with function approximation: Recent advances and applications. Information Sciences 261.1-31. ISSN 0020-0255. doi:org/10.1016/j.ins.2013.08.037.
Comments
Post a Comment